We interact with subjective rating systems on a daily basis. But when accuracy is imperative, it’s important to have an objective risk scoring model to turn to.
One way we work to simplify our complex, data-driven world is to figure out a system to easily summarize and digest the large amounts of information that come our way each day. We have rating systems for everything from restaurants to movies, to cars and even homes. Instead of providing objective ratings for specific characteristics, we often condense subjective opinions into a single score that encapsulates a number of different factors. But how are these ratings or “scores” calculated?
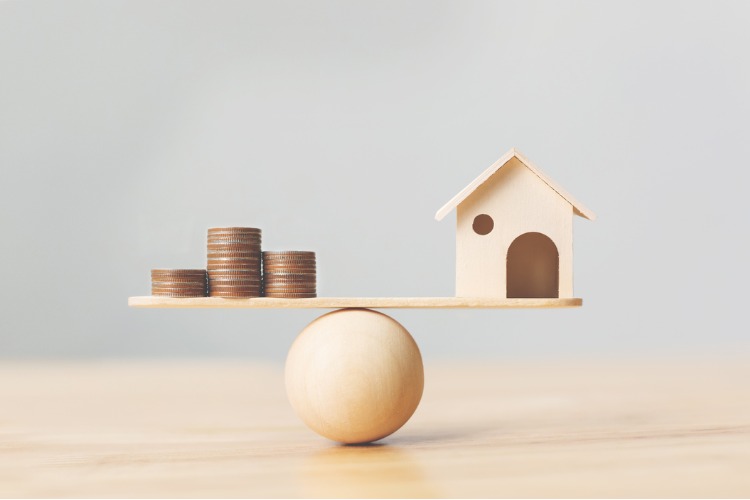
Many times our rating systems are subject to knee-jerk reactions, and they are often skewed by difficult-to-quantify factors such as our feelings on a given day, our bias towards individuals or companies, or even the opinions of our friends and family. Need an example? Just think of any online restaurant reviews you have read recently. With data sets that require objective analysis, however, there needs to be a way to limit bias from these ratings/scores in order to develop a consistent and easy risk scoring method.
What Are Weighted Risk Scoring Models?
Essentially, think of weighted scoring models as indexes consisting of pre-established criteria. Based on what the score is trying to communicate, each criterion is given a unique weight, which will determine the level of the criterion’s influence on the overall score. Often, these models are applied in the financial services or property & casualty insurance industries to determine risk levels for a given feature (e.g. home, neighborhood, individual).
Risk scoring models and the statistics that drive them are highly important because they establish a consistent, calculated way to evaluate risk. Without a scientific method to measure the probability of say, a flood occurring in a specific location, it would become exceedingly difficult for companies insuring homes to confidently build their portfolios to a tolerable level of risk.
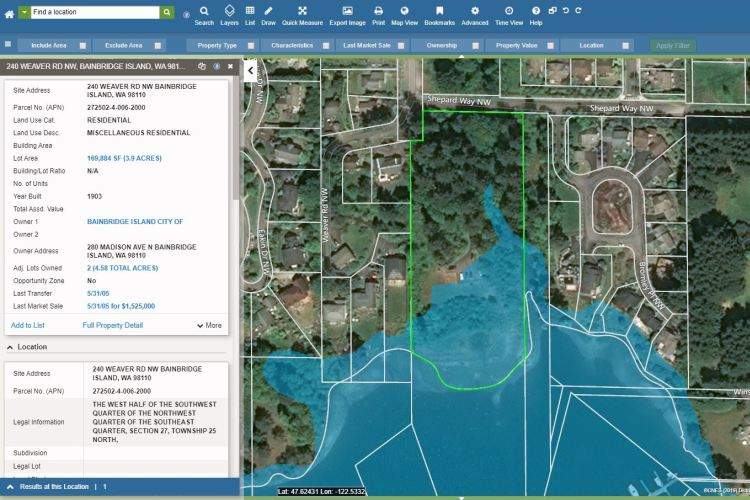
You can apply this same concept to ratings such as “walkability” scores as well. These are often used to communicate to prospective buyers a home’s relative proximity to important features (e.g. schools, transit, shopping centers, etc.). The higher the score, the “better” the neighborhood.
In order to fully grasp the idea of weighted scoring, let’s try to take this concept and apply it to our own neighborhood score below:
We can start by selecting the criteria that we will use to build the score (what traits would make a neighborhood more livable?):
- Proximity to Schools
- The closer the home is to the school, the better
- Proximity to Public Transit
- Being close to public transit is important because it allows easy access to many different amenities
- Crime
- Needless to say, it is very important to be in an area free of crime
Based on the above information, the next step is to assign weights to each criterion (Note: the weights should add up to 100%):
- Proximity to schools: 25%
- Proximity to public transit: 30%
- Crime level: 45%
In the above, we have chosen “crime level” as our most important factor, “proximity to public transit” as our second-most, and “proximity to schools” as the factor we consider least important of the three. Now that the weights have been determined, we need to assign a raw value/score to each of the criterion (such as distance intervals):
25% Weight
Distance to nearest school (miles) | | Raw Score |
0-1 | 5 |
1-2 | 4 |
2-3 | 3 |
3-4 | 2 |
4+ | 1 |
30% Weight
Distance to nearest bus stop (miles) | | Raw Score |
0-0.5 | 5 |
0.5-1 | 4 |
1-1.5 | 3 |
1.5-2 | 2 |
2+ | 1 |
45% Weight
Crime ratings | | Raw Score |
0-10 (very low) | 5 |
10-30 (below average) | 4 |
30-50 (average) | 3 |
50-100 (high) | 2 |
100+ (very high) | 1 |
Now, let’s say the home in question is:
- 2.2 miles from the nearest school
- 0.3 miles from the nearest bus stop
- Located in an area with a high crime rate
If we substitute the raw score value for each criterion into the below equation, we achieve an overall score for the home. 3(0.25) + 5(0.3) + 2(0.45) = 3.15 out of 5
Depending on the organization’s/individual’s tolerance, a score of 3.15 maybe be considered either below or above average – this is totally dependent on the situation.
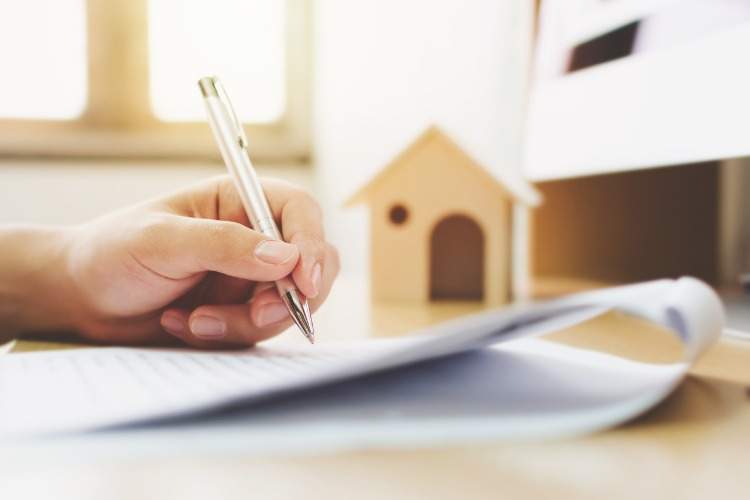
Obviously, the above example is oversimplified. Organizations undergo multiple iterations of score generations, with various combinations of criteria, in order to come up with the most meaningful score to them. For example, when evaluating the neighborhood score of a home, a different company may consider proximity to schools to be more important than the crime level. They would then modify the weights accordingly to tailor the score to suit their needs.
Often, the task of creating these scores is outsourced to a third party. In some cases, companies may lack the expertise to build these models or simply not have access to the data (criteria) required to produce a meaningful score. More and more, location technology and data providers are being employed to build models based on client requirements.
Why Should You Use a Weighted Risk Scoring Model?
The concept of weighted risk scoring is a relatively simple one, and these models bring many benefits to your analysis:
- Weighted models are often highly customizable
- Weighted models are consistent and have limited bias (obviously there may be some bias based on who is actually building the score; for example, one person may believe that proximity to schools is more important than proximity to bus stops)
- Weighted models can be applied across very large datasets. For example, a company can run a weighted risk model on its entire portfolio and can expect a consistent and measurable result across the board
- Weighted models can quickly provide a meaningful score based on many different types of input data from which educated business decisions can be made
Learn More
If you would like to learn how property and location data can be used to generate weighted scoring models, contact us today!
Author Bio
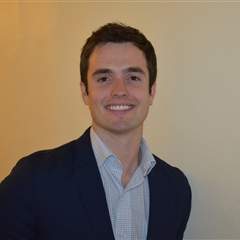
Andrew LeMay is an Associate Product Manager at DMP in their Canadian office. With a background in geospatial data management, he served as a data engineer and GIS specialist before joining the product team. He has recently taken an interest in the field of data science and enjoys learning about how predictive models and simple automation can change the way we think about our daily tasks and routines. On top of that, he is an avid ice hockey fan and loves playing sports when he is not in the office. Contact Andrew at alemay@dmtispatial.com or via LinkedIn.